Setting Up and Using Jupyter Labs with Docker Compose
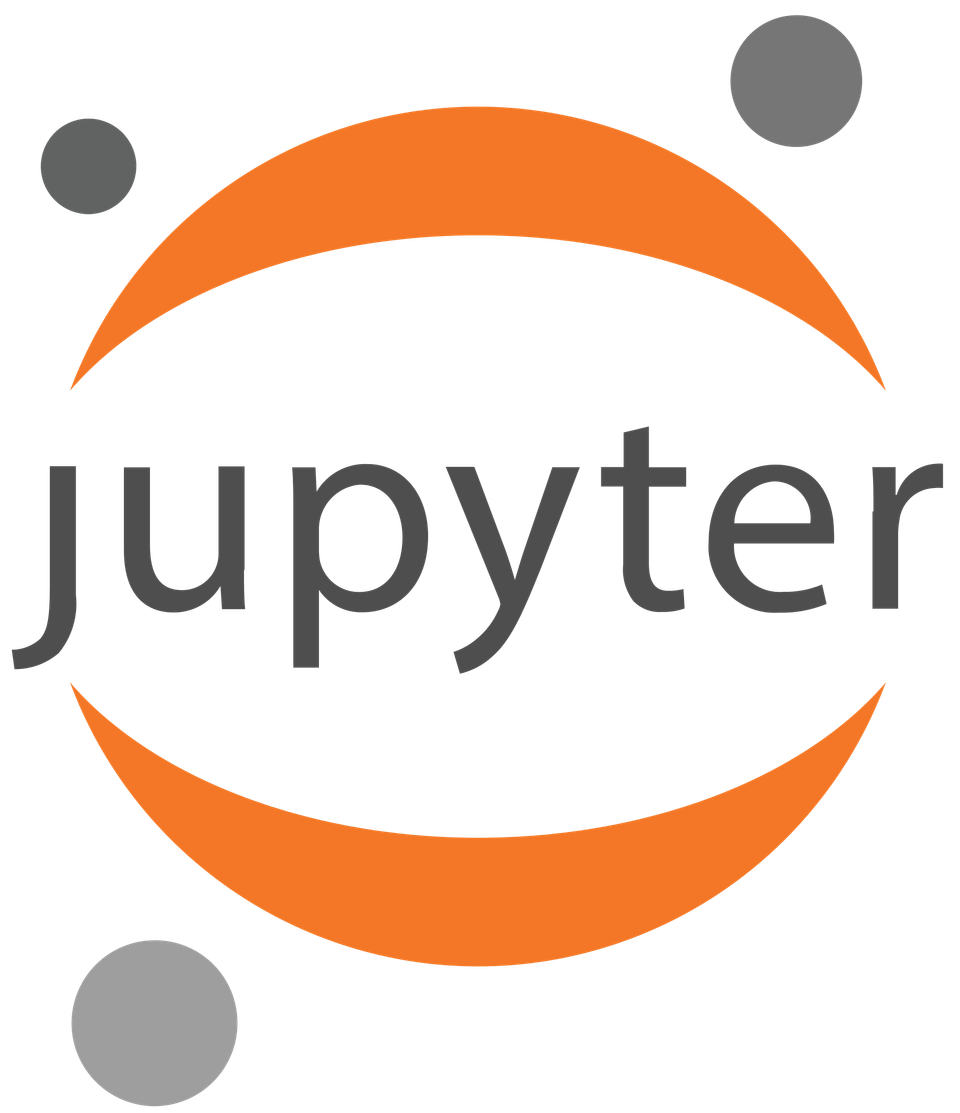
Introduction
Jupyter Lab is an essential tool for data scientists, providing an interactive environment for running notebooks, code, and data visualizations. Setting it up with Docker Compose ensures a consistent, isolated environment, leveraging GPU resources for enhanced performance.
Note that using the GPU version of Jupyter labs is not required, but I am using it for running ML programs and processing on CPU would be far slower.
Why Jupyter Labs?
1. Rapid Iteration:
Jupyter Lab enables quick prototyping and experimentation. By providing an interactive coding environment, you can write and test code snippets, visualize data, and see results in real-time, making it ideal for data science workflows.
2. Interactive Visualizations:
With built-in support for rich media outputs, Jupyter Lab is perfect for data visualization. It supports libraries like Matplotlib, Seaborn, and Plotly, allowing you to create and display charts, graphs, and other visualizations directly in your notebook.
3. Ease of Use:
The intuitive interface of Jupyter Lab makes it accessible to both beginners and experienced users. It supports multiple programming languages via kernels, though Python is the most commonly used.
4. Enhanced Collaboration:
Jupyter Lab's ability to share notebooks and work collaboratively with others is a significant advantage. Notebooks can be exported in various formats, including HTML and PDF, and shared with colleagues for review and collaboration.
You can find my Jupyter Notebooks here: https://github.com/cskujawa/jupyter-notebooks/tree/main
5. GPU Acceleration:
Leveraging GPUs in data science tasks can significantly speed up computations, especially for machine learning and deep learning applications. The Docker setup ensures that your Jupyter Lab environment is optimized for GPU usage, providing a performance boost for intensive tasks.
Docker Compose Definition
Here's the Docker Compose configuration I used for setting up my instance of Jupyter Lab:
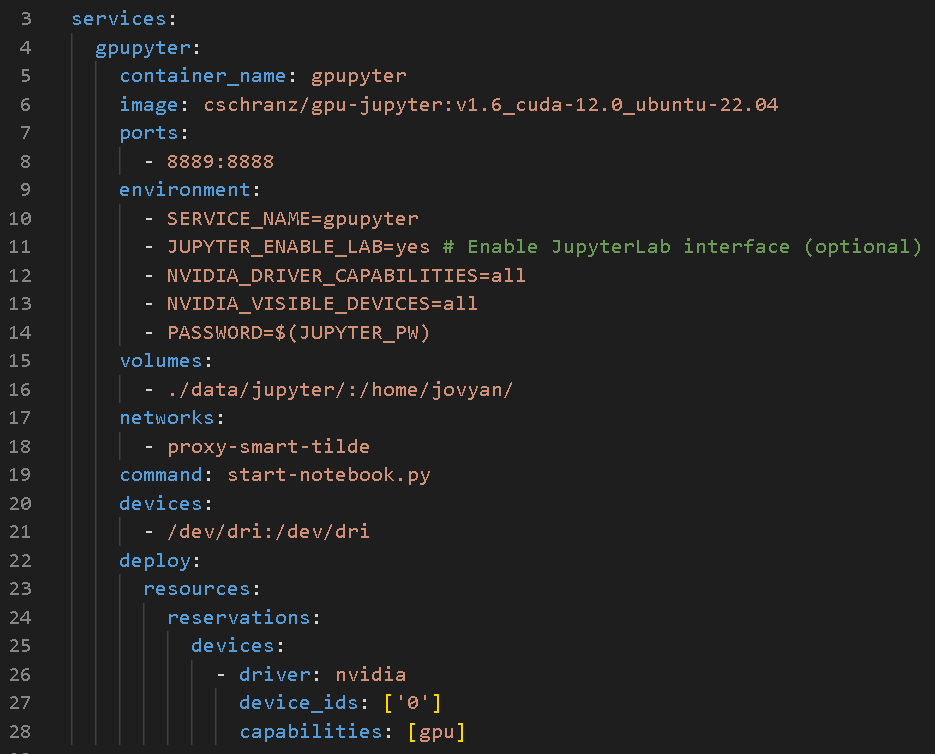
Explanation
- Container Configuration:
- Image: Uses
cschranz/gpu-jupyter:v1.6_cuda-12.0_ubuntu-22.04
, which is tailored for GPU support with CUDA 12.0 and Ubuntu 22.04.
- Image: Uses
- Environment Variables:
- JUPYTER_ENABLE_LAB: Enables the Jupyter Lab interface.
- NVIDIA_DRIVER_CAPABILITIES and NVIDIA_VISIBLE_DEVICES: Required for GPU support.
- PASSWORD: Sets the password for Jupyter Lab access.
- Volumes:
- Maps the host directory
./data/jupyter/
to the container's/home/jovyan/
to persist notebooks and data.
- Maps the host directory
- Command:
- Runs
start-notebook.py
to initiate the Jupyter Lab server.
- Runs
- Devices:
- Maps
/dev/dri
to allow GPU access within the container.
- Maps
- Deployment Resources:
- Reserves GPU resources by specifying the NVIDIA driver, device IDs, and capabilities.
A guide for using Jupyter labs is out of scope for this blog post, but Jupyter has great documentation available here:
https://jupyter-notebook.readthedocs.io/en/stable/
I've been working in my Jupyter Lab for a few months now and it has been a fantastic experience. I really wanted to set up the Jupyter Lab so I could test HuggingFace models out, try different projects, and not have to worry about building a whole app every time.
I'll be writing some future blog posts on the different projects I've tackled using it.
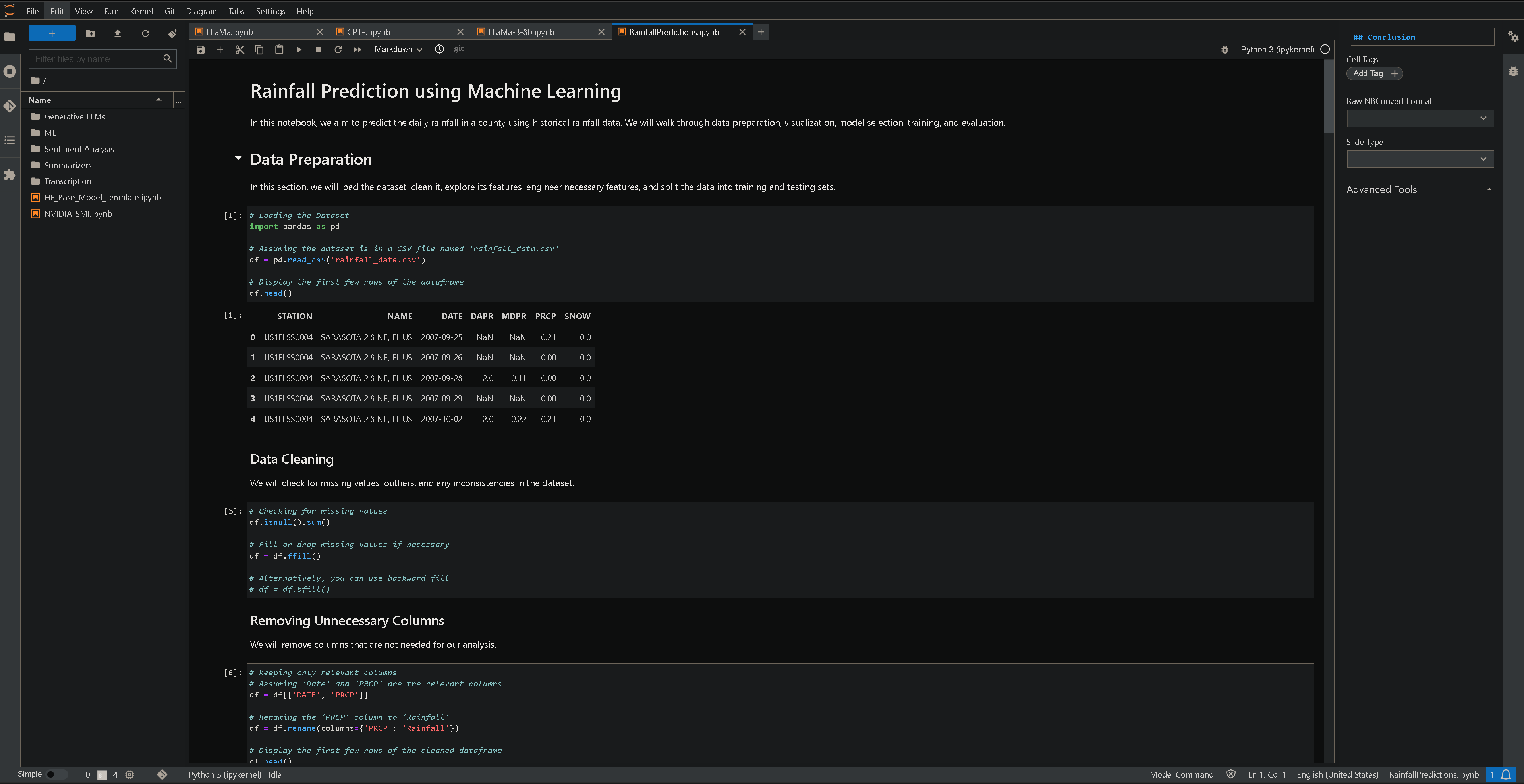
Future Topics
Stay tuned for future blog posts where we will use Jupyter Labs to delve into:
- Generative LLMs: Exploring generative language models.
- Machine Learning: Notebooks on predicting using machine learning techniques.
- Sentiment Analysis: Projects on analyzing sentiment in text data.
- Text Summarization: Notebooks for summarizing text.
- Transcription: Projects related to transcribing audio data.
Conclusion
Setting up Jupyter Lab with Docker Compose streamlines the deployment process, especially when leveraging GPU resources. This setup ensures a consistent, isolated environment, enhancing reproducibility and performance for data science tasks. Whether you're prototyping machine learning models or visualizing complex datasets, Jupyter Lab offers a powerful and flexible platform to support your work. Happy coding!