Getting Started with Pretrained Models from Hugging Face in Jupyter Lab
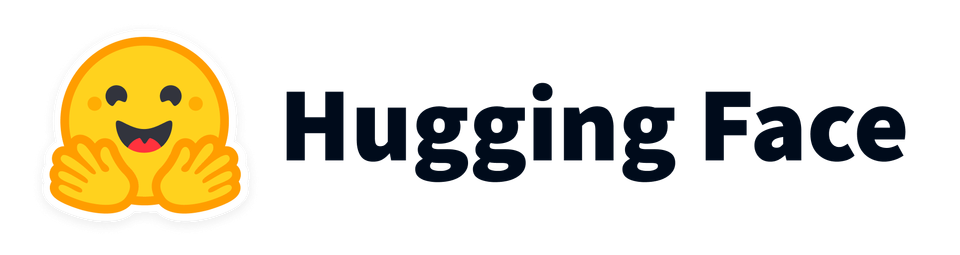
Today we're going to be getting started with pretrained models from Hugging Face. If you're new to the world of machine learning or just looking to explore how to use powerful pretrained models, you're in the right place. We'll be running our project in Jupyter Lab, so make sure you have it installed and ready to go, if not you can check out my post on Jupyter Lab here. Let's dive in!
What is Hugging Face?
Hugging Face is a company that has made a significant impact in the field of natural language processing (NLP). They offer a library called transformers
, which provides access to a wide variety of pretrained models for tasks like text classification, question answering, translation, and much more. These models are trained on massive datasets and can save you a ton of time and resources.
Installing the Transformers Library and Dependencies
Before we can use Hugging Face's models, we need to install the transformers
library and any necessary dependencies. Open a new terminal in Jupyter Lab and run:
# Install transformers dependencies
!pip install -qU transformers
!pip install accelerate
# Had to add in the install tensorflow (which should already be installed in this container) with the [and-cuda] to get some extra functions out of it
!pip install tensorflow[and-cuda]
import transformers
This will install the library and its dependencies, allowing us to access and use the pretrained models.
Signing in to Hugging Face
Before we can access Hugging Face's models we'll need to login. You'll need your authentication information from your Hugging Face account, I use the authentication token. You can find yours here:
https://huggingface.co/settings/tokens
# Install Huggingface dependency and login using token
!pip install --upgrade huggingface_hub
from huggingface_hub import login
login()
Loading a Pretrained Model
Now, let's load a pretrained model. For this example, we'll use a sentiment analysis model to classify text as positive or negative. We'll use the pipeline
API, which makes it easy to use pretrained models for various tasks.
First, let's import the necessary libraries and set up our pipeline:
from transformers import pipeline
# Load a sentiment analysis pipeline
classifier = pipeline('sentiment-analysis')
The pipeline
function abstracts away the complexities of loading models and tokenizers. By specifying sentiment-analysis
, we're loading a pipeline tailored for this specific task.
Performing Sentiment Analysis
With our pipeline set up, we can now perform sentiment analysis on some sample text. Let's see how it works:
# Define some text to analyze
text = "I absolutely love the new features in the latest update! It's amazing!"
# Use the classifier to analyze the sentiment
result = classifier(text)
# Print the result
print(result)
When you run this code, you'll see the model's prediction. In this case, it will likely classify the text as positive. The output will look something like this:
[{'label': 'POSITIVE', 'score': 0.9998745918273926}]
The label
indicates the predicted sentiment, and the score
represents the confidence level of the prediction.
Exploring More Use Cases
The transformers
library supports a wide range of tasks beyond sentiment analysis. Here are a few examples:
Text Generation:
generator = pipeline('text-generation', model='gpt-2')
result = generator("Once upon a time", max_length=50, num_return_sequences=1)
print(result)
Question Answering:
question_answerer = pipeline('question-answering')
context = "Hugging Face Inc. is a company based in New York City. Its headquarters are in DUMBO, therefore very close to the Manhattan Bridge."
question = "Where is Hugging Face based?"
result = question_answerer(question=question, context=context)
print(result)
Translation:
translator = pipeline('translation_en_to_fr')
result = translator("Hello, how are you?")
print(result)
Conclusion
And there you have it! We've successfully used a pretrained model from Hugging Face to perform sentiment analysis in Jupyter Lab. Hugging Face's transformers
library makes it incredibly easy to access and use powerful models for a variety of NLP tasks. Whether you're analyzing sentiment, generating text, answering questions, or translating languages, the possibilities are endless.
I hope this guide helps you get started with pretrained models from Hugging Face. Happy coding, and feel free to share your experiences and any cool projects you create using these amazing tools!
Happy coding!