Computer Vision / Streamlit / Memory Management
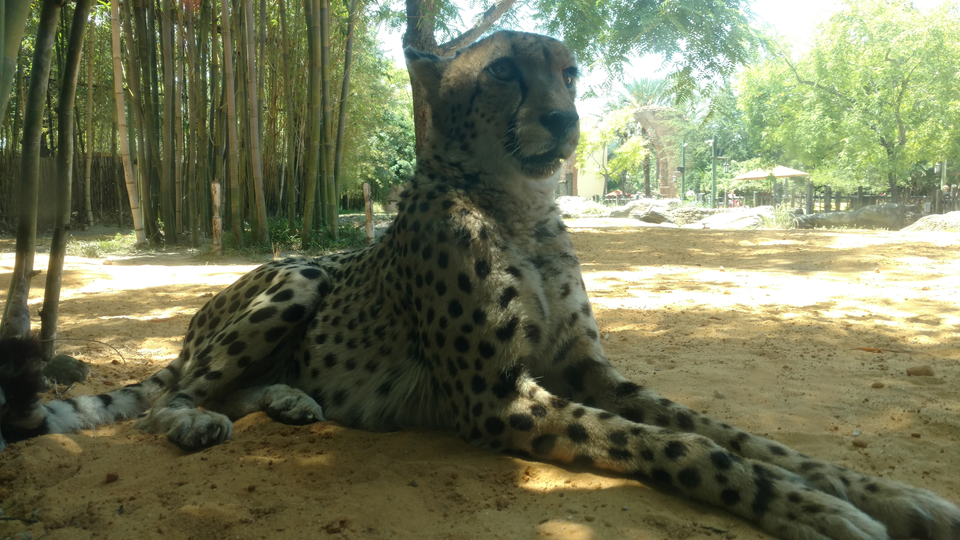
While working on this project I learned a lot about the different models used for Computer Vision. I also got a grip on Streamlit which is a pure python front end, I've already really started to like Streamlit for it's simplicity, although I won't be covering Streamlit in this post.
Along the way I ran into some issues with RAM usage and learned some new ways to prevent excessive memory usage and proper methods for releasing RAM after using models. One issue I was not able to overcome was with larger LLMs, I simply did not have enough RAM to load them into memory to use them. I ended up opting for OpenAIs API to accomplish the generative text portion of the project. GPT2 did load but it's performance was abysmal.
RAM Usage
I only figured out two ways to free RAM, I needed to either delete the variable or free up the Torch resource (which I was not using in this project.)
# Clear memory
del model
#torch.cuda.empty_cache() if torch.cuda.is_available() else None
Now, my server isn't exactly light on RAM, it hosts a lot of different applications and services, but it has a solid 32GB of EEC RAM. It's not a huge quantity, but it has served me well up to this point. When it comes to LLMs though, it's not enough. Below is a screenshot of Netdata with the usage stats at idle.
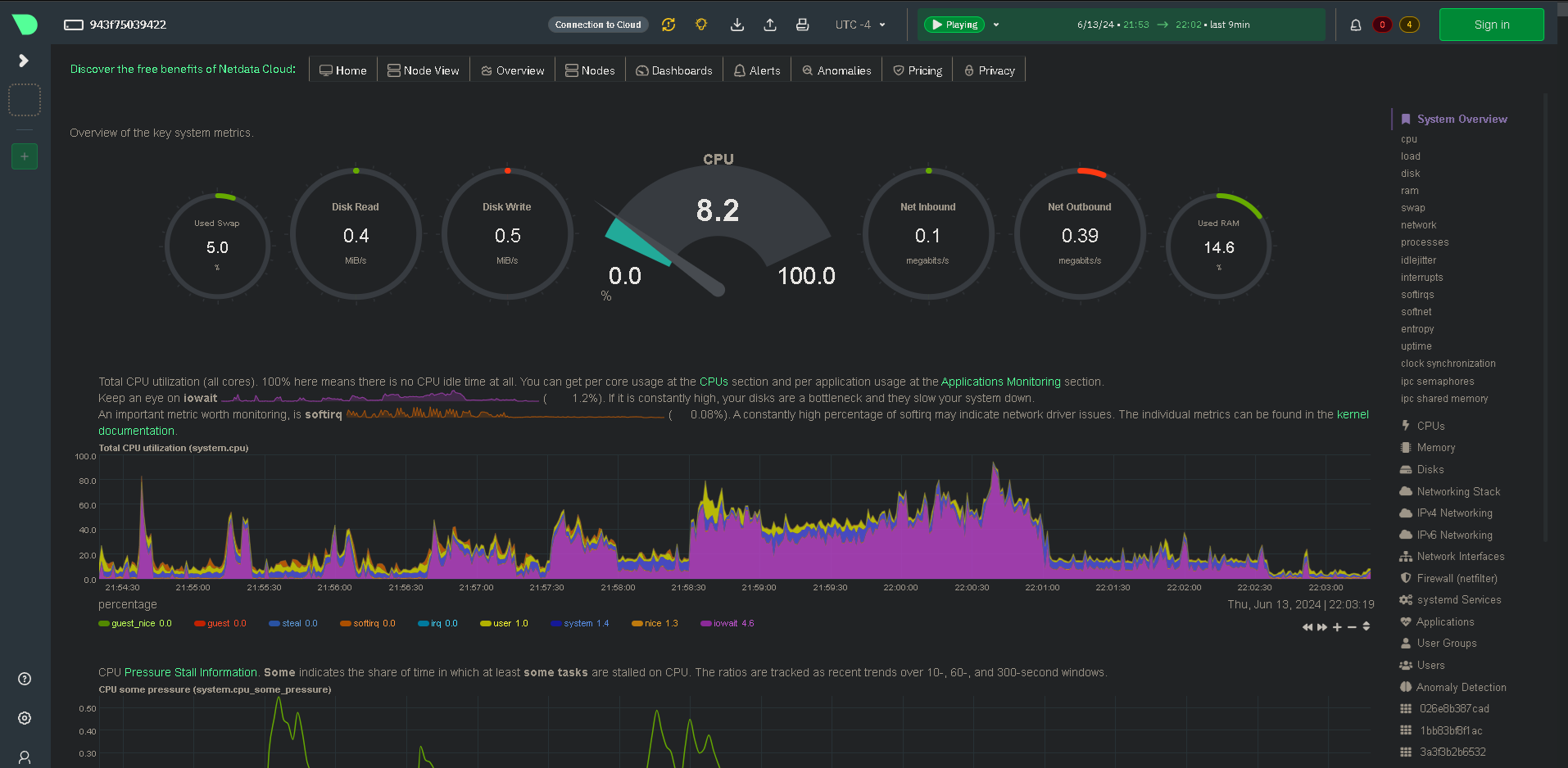
Below is a screenshot of the same usage stats after starting to load an LLM via transformers.
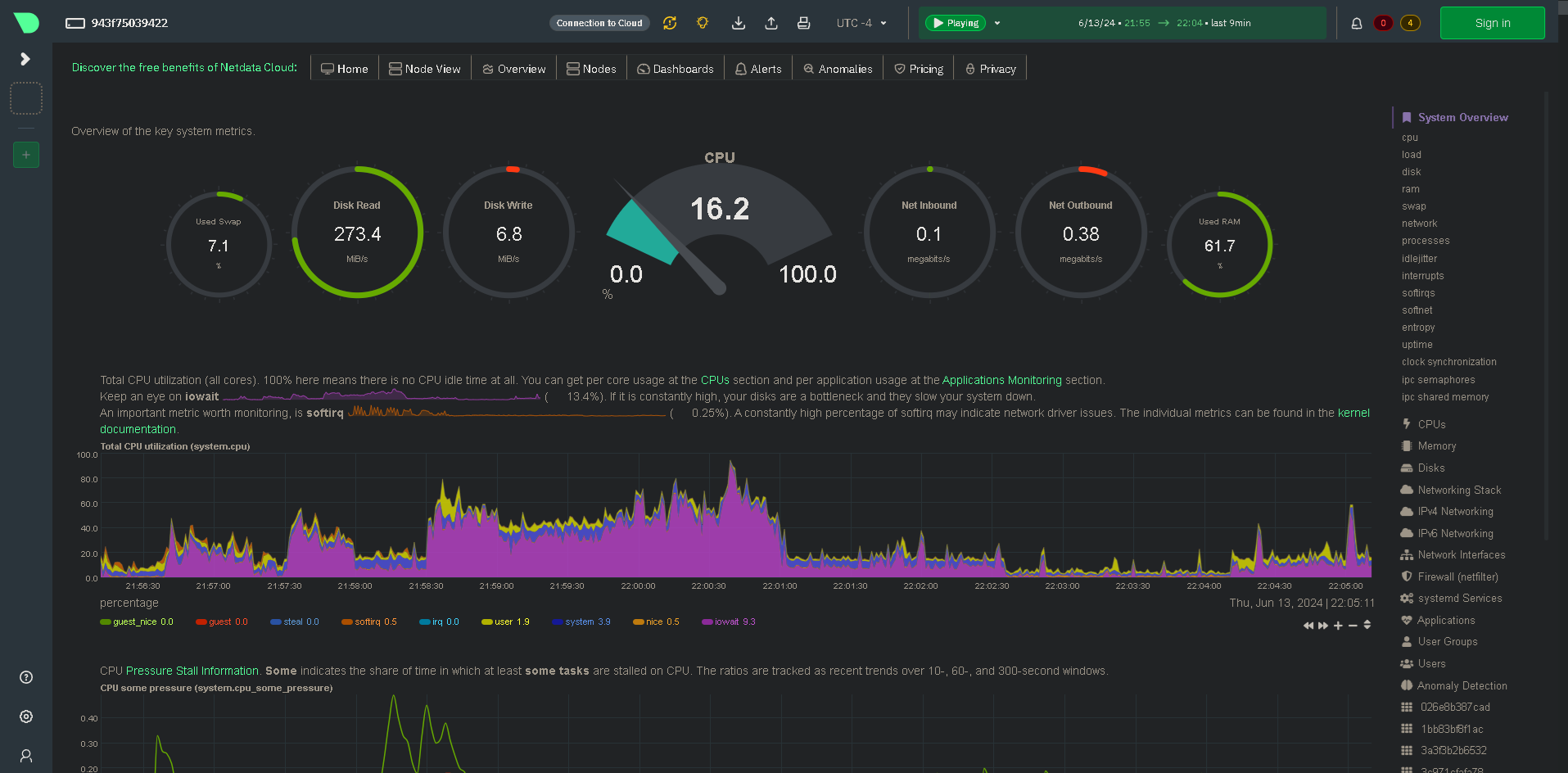
Lastly, the usage stats just before the server crashed. Nearly 100% RAM usage and 90% swap usage, not good.
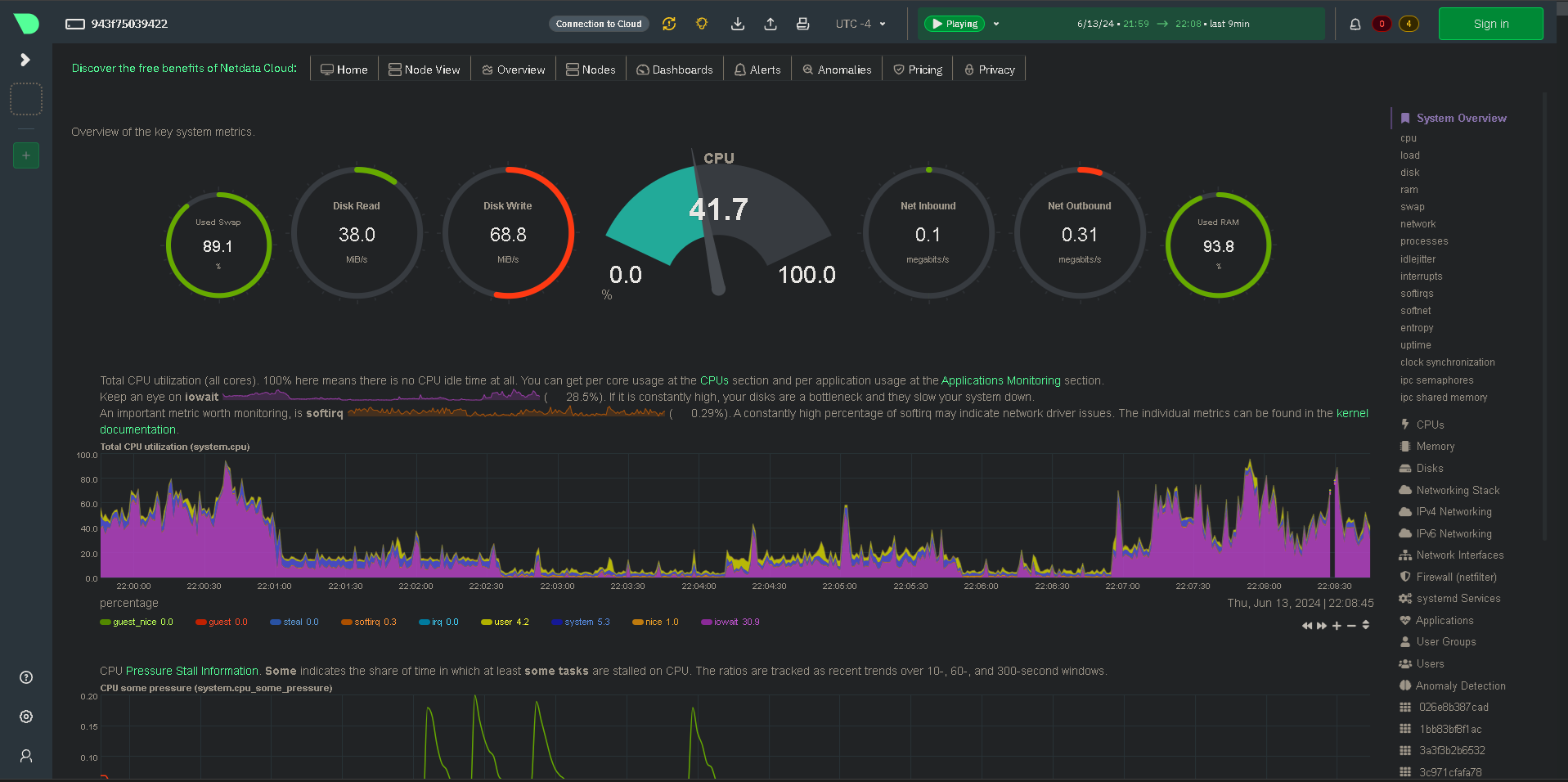
Ultimately I got it to work using the OpenAI API for text generation instead of a locally hosted LLM. That's okay, in the future I'll get it sorted out. Regardless of the text generation though, I got the rest of the models loaded in and working. It was pretty easy too when all the models I was testing with were in the Tensorflow Keras Applications package.
from tensorflow.keras.applications.efficientnet import EfficientNetB0, preprocess_input as efficientnet_preprocess_input, decode_predictions as efficientnet_decode_predictions
from tensorflow.keras.applications.resnet50 import ResNet50, preprocess_input as resnet_preprocess_input, decode_predictions as resnet_decode_predictions
from tensorflow.keras.applications.inception_v3 import InceptionV3, preprocess_input as inception_preprocess_input, decode_predictions as inception_decode_predictions
from tensorflow.keras.applications.mobilenet import MobileNet, preprocess_input as mobilenet_preprocess_input, decode_predictions as mobilenet_decode_predictions
from tensorflow.keras.applications.densenet import DenseNet121, preprocess_input as densenet_preprocess_input, decode_predictions as densenet_decode_predictions
from tensorflow.keras.applications.vgg16 import VGG16, preprocess_input as vgg16_preprocess_input, decode_predictions as vgg16_decode_predictions
Now getting them all loaded in was fine and using Streamlit to pull together a UI made it easy. To actually glean some insight from the application though I made some changes. First of all I made it so all the results from each model were placed in the same table, and then I sorted the table by the probability (the confidence.) So whichever model was most confident was at the top with it's guess. The models aren't perfect, but at some tasks they excelled.
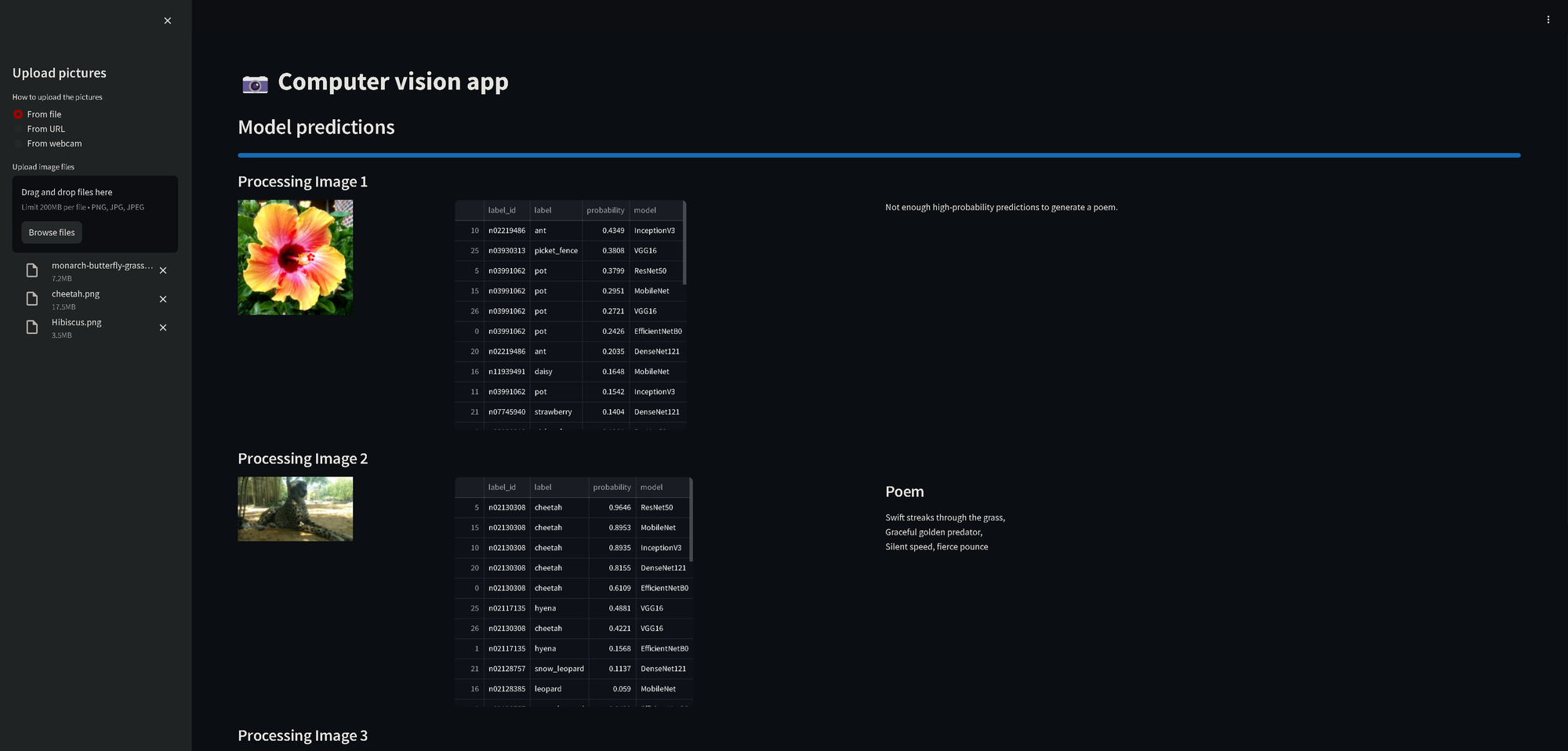
With a little bit of tweaking and setting some thresholds, I got it to write a poem about the image it saw, that is if at least a few of the models had more than 50% probability in their guesses.