Breaking Down AI - Understanding Convolutional Neural Networks
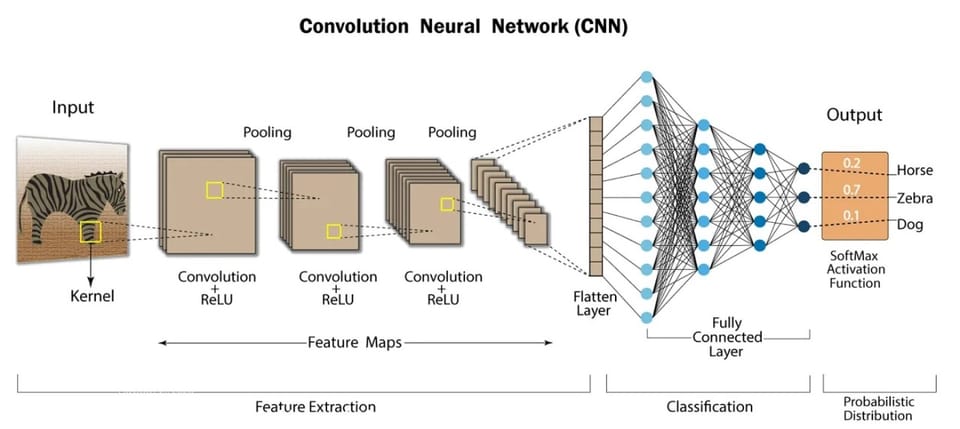
Convolutional Neural Networks (CNNs) have revolutionized the field of computer vision and deep learning, becoming a cornerstone technology for image processing and recognition tasks. In this blog post, we will explore the origins of CNNs, delve into how they work, and discuss why they are so widely used across various applications.
Origins of CNNs
The concept of Convolutional Neural Networks traces back to the 1980s when Kunihiko Fukushima introduced the "Neocognitron," a model designed for pattern recognition.
The Neocognitron was inspired by the model proposed by Hubel & Wiesel in 1959. They found two types of cells in the visual primary cortex called simple cell and complex cell, and also proposed a cascading model of these two types of cells for use in pattern recognition tasks.
However, it wasn't until the 1990s that CNNs gained significant traction, largely due to the pioneering work of Yann LeCun and his colleagues. They developed the LeNet architecture, which was successfully used for digit recognition in zip codes, showcasing the practical potential of CNNs.
How CNNs Work
CNNs are specially designed for processing grid-like data, such as images. Here's a breakdown of their key components and how they function:
- Convolutional Layers:
Convolutional layers apply convolution operations to the input data. A convolution operation involves a filter (or kernel) that slides over the input data, performing element-wise multiplication and summation to produce a feature map. These filters are learnable parameters, enabling the network to identify essential features such as edges, textures, and patterns. - Pooling Layers:
Pooling layers reduce the spatial dimensions of the feature maps, making computations more efficient and reducing the risk of overfitting. Common pooling operations include max pooling (selecting the maximum value within a window) and average pooling (computing the average value within a window). - Activation Functions:
Non-linear activation functions, such as ReLU (Rectified Linear Unit), introduce non-linearity into the model, allowing it to learn more complex patterns. - Fully Connected Layers:
After several convolutional and pooling layers, the feature maps are flattened and fed into fully connected (dense) layers. These layers function like traditional neural networks, where each neuron is connected to every neuron in the previous layer. - Output Layer:
The final layer produces the output, which can be a classification (e.g., softmax for multi-class classification) or regression (e.g., linear activation for continuous values).
Why CNNs Are Used
CNNs are popular for several reasons:
- Spatial Hierarchy of Features:
CNNs effectively capture spatial hierarchies in images, from low-level features (like edges) to high-level features (like objects). This hierarchical learning enables robust feature extraction. - Parameter Sharing:
By using the same filter across different parts of the input, CNNs significantly reduce the number of parameters compared to fully connected networks, making them more efficient and less prone to overfitting. - Translation Invariance:
CNNs are inherently translation-invariant, meaning they can recognize objects regardless of their position in the image. This property is crucial for tasks like object detection and image classification. - Versatility:
While originally designed for image data, CNNs have been successfully applied to various types of data, including time-series data, audio signals, and even text (in the form of character-level or word-level embeddings). - Performance:
CNNs have achieved state-of-the-art performance in many computer vision tasks, including image classification (e.g., AlexNet, VGG, ResNet), object detection (e.g., YOLO, Faster R-CNN), and image segmentation (e.g., U-Net).
Applications of CNNs
CNNs are used in a wide range of applications:
- Image Classification: Identifying objects within images (e.g., ImageNet competition).
- Object Detection: Locating and classifying objects within an image (e.g., self-driving cars).
- Image Segmentation: Dividing an image into segments for detailed analysis (e.g., medical imaging).
- Face Recognition: Identifying and verifying individuals' faces (e.g., security systems).
- Natural Language Processing: Applying CNNs to text data for tasks like sentiment analysis and language modeling.
Conclusion
Convolutional Neural Networks are a powerful and versatile tool in deep learning, particularly suited for tasks involving visual data and other structured grid-like data. Their ability to automatically and adaptively learn spatial hierarchies of features makes them invaluable for a wide range of applications. As the field of deep learning continues to evolve, CNNs will undoubtedly remain at the forefront of technological advancements.